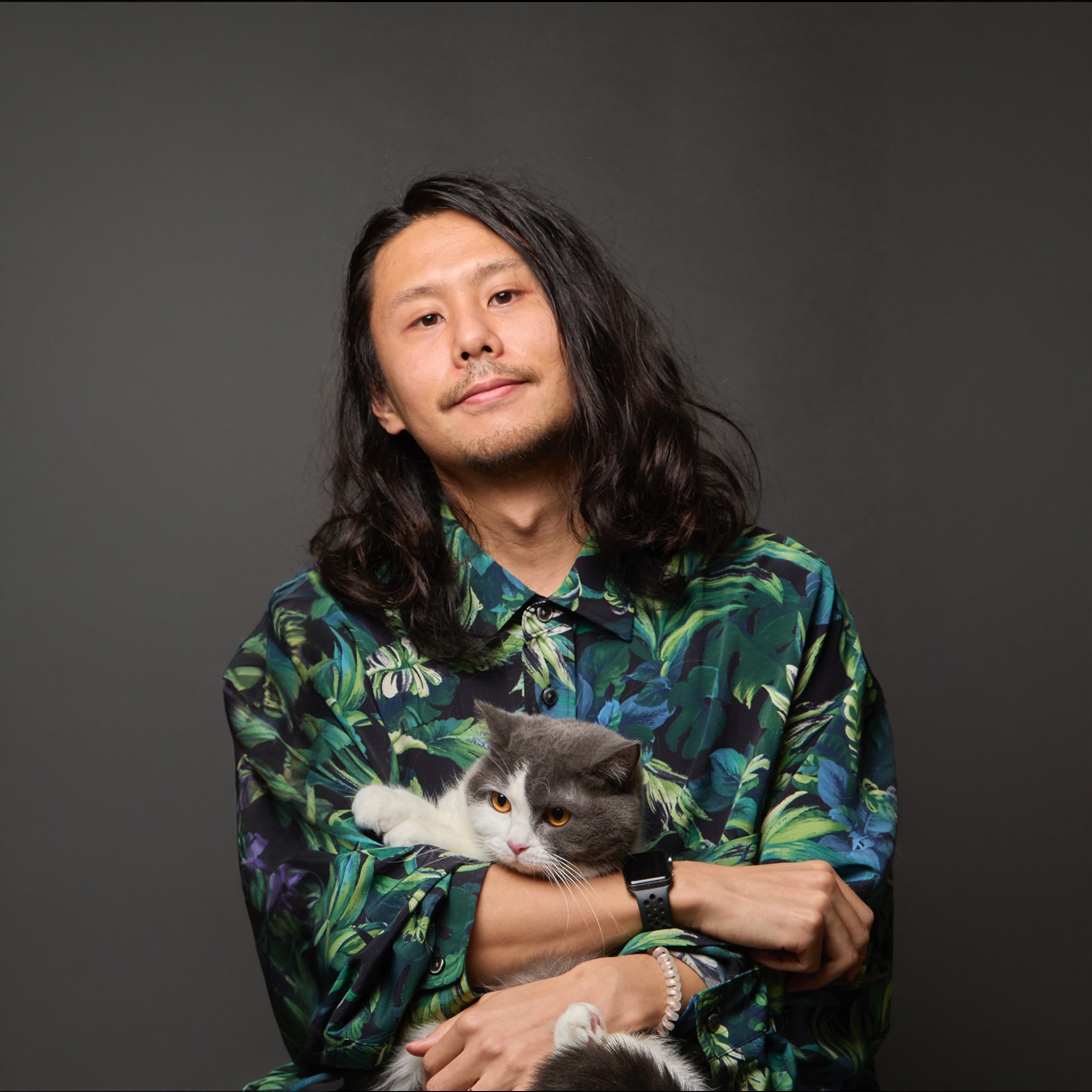
Shin'ya Yamaguchi
I am an associate distinguished researcher at NTT and a Ph.D student at Kyoto University (Kashima Lab.). My research interests are machine learning with synthetic data, generative models, vision-language models, explainability, distribution shifts, self-supervised learning, and semi-supervised learning.
Updates
- [2025/2/26] Our paper Post-pre-training for Modality Alignment in Vision-Language Foundation Models has been accepted to CVPR 2025! We propose a very lightweight post-pre-training method for aligning pre-trained vision-language models like CLIP. Stay tuned for more details!
- [2025/01/22] Our paper Test-time Adaptation for Regression by Subspace Alignment has been accepted to ICLR 2025! This paper proposes a novel test-time adaptation method for regression tasks by aligning features on sub-space.
- [2025/01/21] Our paper Transfer Learning with Pre-trained Conditional Generative Models has been accepted to Machine Learning Journal (ECML-PKDD Journal Track)! We propose a generative transfer learning method based on pre-trained large generative models for a severe setting where we cannot access source datasets and pre-trained weights for target tasks.
- [2024/12/10] Our paper Explanation Bottleneck Models has been accepted to AAAI 2025 (acceptance rate: 23%)! We propose a new interpretable model that generates text explanations and predicts the final label based on the text explanation. This work will be presented at the Workshop on Foundation Model Intervention (MINT) @ NeurIPS2024!
- [2024/9/5] My solo paper Analyzing Diffusion Models on Synthesizing Training Datasets and co-authored paper Toward Data Efficient Model Merging between Different Datasets without Performance Degradation have been accepted to ACML 2024 (acceptance rate: 20%)!
- [2024/4/1] I’m happy to announce that I have been promoted to associate distinguished researcher at NTT!
- [2024/3/18] Our two papers Test-Time Similarity Modification for Person Re-Identification Toward Temporal Distribution Shift and Test-Time Adaptation Meets Image Enhancement: Improving Accuracy via Uncertainty-Aware Logit Switching have been accepted to IJCNN 2024!
Past Updates
- [2024/2/26] Our paper Adaptive Random Feature Regularization on Fine-tuning Deep Neural Networks has been accepted to CVPR 2024! We propose a simple yet effective fine-tuning method by penalizing feature extractors with random reference vectors generated from adaptive class-conditional priors.
- [2023/11/23] Our preprint On the Limitation of Diffusion Models for Synthesizing Training Datasets appeared in arXiv! We analyzed diffusion models with various perspectives and found that modern diffusion models have a limitation on the ability to replicate datasets in terms of accuracy when the synthetic samples are used for training classifiers. This work will be presented at NeurIPS 2023 SyntheticData4ML Workshop.
- [2023/11/15] My solo paper Generative Semi-supervised Learning with Meta-Optimized Synthetic Samples has been received Best Paper Award from ACML 2023!
- [2023/09/22] Our paper Regularizing Neural Networks with Meta-Learning Generative Models has been accepted to NeurIPS 2023! In this paper, we propose a novel meta-learning-based regularization method (MGR) using synthetic samples from pre-trained generative models. In contrast to conventional generative data augmentation methods, MGR utilizes synthetic samples for regularizing only feature extractors and finds useful samples through meta-learning of latent variables.
- [2023/09/11] My solo paper Generative Semi-supervised Learning with Meta-Optimized Synthetic Samples has been accepted to ACML 2023! This paper proposes a real unlabeled-dateless semi-supervised learning that utilizes a foundation generative models as the unlabeled data source. We introduce a meta-optimization-based sampling algorithm for extracting synthetic unlabeled data from the foundation generative model and a cosine similarity-based unsupervised loss function for updating the feature extractor of the classifier by the synthetic samples.
Activities
Services as a Reviewer
- 2022: ICML, NeurIPS
- 2023: CVPR, PAKDD, ICML, ICCV, NeurIPS, IPSJ, DMLR@ICML2023, BMVC, ACML, TNNLS
- 2024: WACV, ICLR, CVPR, DMLR@ICLR2024, ICML, ECCV, NeurIPS, NeurIPS DB Track, ACML, DMLR@ICML2024, TMLR
- 2025: AAAI, ICLR, AISTATS, CVPR, ICML, TMLR, ICCV, NeurIPS
Biography
Apr. 2022 - Current
Ph.D student at Dept. of Intelligence Science & Technology, Graduate School of Informatics, Kyoto University
Apr. 2017 - Current
Researcher at NTT
Apr. 2015 - Mar. 2017
M.E. from Dept. of Computer Engineering, Graduate School of Engineering, Yokohama National University
Apr. 2011 - Mar. 2015
B.E. from Dept. of Computer Engineering, Yokohama National University
Publications
International Conference
- S. Yamaguchi, F. Dewei, S. Kanai, K. Adachi, D. Chijiwa,
Post-pre-training for Modality Alignment in Vision-Language Foundation Models,
The IEEE/CVF Conference on Computer Vision and Pattern Recognition (CVPR), 2025. - K. Adachi, S. Yamaguchi, A. Kumagai,
Test-time Adaptation for Regression by Subspace Alignment,
International Conference on Learning Representations (ICLR), 2025. [OpenReview] - S. Yamaguchi and K. Nishida,
Explanation Bottleneck Models,
AAAI Conference on Artificial Intelligence (AAAI), Oral, 2025. [code] - S. Yamaguchi,
Analyzing Diffusion Models on Synthesizing Training Datasets,
Asian Conference on Machine Learning (ACML), 2024. [OpenReview] - M. Yamada, T. Yamashita, S. Yamaguchi, D. Chijiwa,
Toward Data Efficient Model Merging between Different Datasets without Performance Degradation,
Asian Conference on Machine Learning (ACML), 2024. [arXiv] [OpenReview] - K. Adachi, S. Enomoto, T. Sasaki, S. Yamaguchi,
Test-Time Similarity Modification for Person Re-Identification Toward Temporal Distribution Shift,
International Joint Conference on Neural Networks (IJCNN), 2024. - S. Enomoto, N. Hasegawa, K. Adachi, T. Sasaki, S. Yamaguchi, S. Suzuki, T. Eda,
Test-Time Adaptation Meets Image Enhancement: Improving Accuracy via Uncertainty-Aware Logit Switching,
International Joint Conference on Neural Networks (IJCNN), 2024. - S. Yamaguchi, S. Kanai, K. Adachi, D. Chijiwa,
Adaptive Random Feature Regularization on Fine-tuning Deep Neural Networks,
The IEEE/CVF Conference on Computer Vision and Pattern Recognition (CVPR), 2024. [code] [arXiv] - S. Yamaguchi, S. Kanai, A. Kumagai, D. Chijiwa, H. Kashima,
Regularizing Neural Networks with Meta-Learning Generative Models,
Neural Information Processing Systems (NeurIPS), 2023. - S. Yamaguchi,
Generative Semi-supervised Learning with Meta-Optimized Synthetic Samples,
Asian Conference on Machine Learning (ACML), Best Paper Award, 2023. - S. Suzuki, S. Yamaguchi, S. Takeda, S. Kanai, N. Makishima, A. Ando, R. Masumura,
Adversarial Finetuning with Latent Representation Constraint to Mitigate Accuracy-Robustness Tradeoff,
The IEEE/CVF International Conference on Computer Vision (ICCV), 2023. - K. Adachi, S. Yamaguchi, A. Kumagai,
Covariance-aware Feature Alignment with Pre-computed Source Statistics for Test-time Adaptation,
IEEE International Conference on Image Processing (ICIP), 2023. - S. Kanai, S. Yamaguchi, M. Yamada, H. Takahashi, Y. Ida,
Switching One-Versus-the-Rest Loss to Increase the Margin of Logits for Adversarial Robustness,
International Conference on Machine Learning (ICML), 2023. - D. Chijiwa, S. Yamaguchi, A. Kumagai, Y. Ida,
Meta-ticket: Finding optimal subnetworks for few-shot learning within randomly initialized neural networks,
Neural Information Processing Systems (NeurIPS), 2022. - K. Adachi, S. Yamaguchi,
Learning Robust Convolutional Neural Networks with Relevant Feature Focusing via Explanations,
IEEE International Conference on Multimedia & Expo (ICME), 2022. - D. Chijiwa, S. Yamaguchi, Y. Ida, K. Umakoshi, T. Inoue,
Pruning Randomly Initialized Neural Networks with Iterative Randomization,
Neural Information Processing Systems (NeurIPS, Spotlight), 2021. [arXiv] [code] - S. Yamaguchi, S. Kanai,
F-Drop&Match: GANs with a Dead Zone in the High-Frequency Domain,
International Conference on Computer Vision (ICCV), 2021. [arXiv] - S. Yamaguchi, S. Kanai, T. Shioda, S. Takeda,
Image Enhanced Rotation Prediction for Self-Supervised Learning,
IEEE International Conference on Image Processing (ICIP), 2021. [arXiv] - S. Kanai, M. Yamada, S. Yamaguchi, H. Takahashi, Y. Ida,
Constraining Logits by Bounded Function for Adversarial Robustness,
International Joint Conference on Neural Networks (IJCNN), 2021. [arXiv] - S. Yamaguchi, S. Kanai, T. Eda,
Effective Data Augmentation with Multi-Domain Learning GANs,
AAAI Conference on Artificial Intelligence (AAAI), 2020. [arXiv] - S. Yamaguchi, K. Kuramitsu,
A Fusion Techniques of Schema and Syntax Rules for Validating Open Data,
Asian Conference on Intelligent Information and Database Systems (ACIIDS), 2017
International Journal
- S. Yamaguchi, S. Kanai, A. Kumagai, D. Chijiwa, H. Kashima,
Transfer Learning with Pre-trained Conditional Generative Models,
Machine Learning Journal (ECML-PKDD Journal Track), 2025. - T. Sasaki, A. S. Walmsley, S. Enomoto, K. Adachi, S. Yamaguchi
Key Factors Determining the Required Number of Training Images in Person Re-Identification,
IEEE Access.
International Workshop (Refereed)
- S. Yamaguchi and K. Nishida,
Toward Explanation Bottleneck Models,
NeurIPS Workshop on Foundation Model Interventions (MINT), 2024. - K. Adachi, S. Yamaguchi, Atsutoshi Kumagai
Test-time Adaptation for Regression by Subspace Alignment,
The 1st Workshop on Test-Time Adaptation at CVPR 2024. Special Mentioned. - S. Yamaguchi,
Analyzing Diffusion Models on Synthesizing Training Datasets,
Data-centric Machine Learning Workshop at ICLR 2024. - S. Yamaguchi and T. Fukuda,
On the Limitation of Diffusion Models for Synthesizing Training Datasets,
SyntheticData4ML Workshop at NeurIPS 2023. - S. Yamaguchi, S. Kanai, A. Kumagai, D. Chijiwa, H. Kashima,
Regularizing Neural Networks with Meta-Learning Generative Models,
Data-centric Machine Learning Research (DMLR) Workshop at ICML 2023.
Preprints
- S. Kanai, Y. Ida, K. Adachim M. Uchida, T. Yoshida, S. Yamaguchi,
Evaluating Time-Series Training Dataset through Lens of Spectrum in Deep State Space Models,
arXiv, 2024. - S. Yamaguchi, S. Kanai, T. Shioda, S. Takeda,
Multiple pretext-task for self-supervised learning via mixing multiple image transformations,
arXiv, 2019. - K. Kuramitsu, S. Yamaguchi,
XML Schema Validation using Parsing Expression Grammars,
PeerJ PrePrints, 2015
Honors
- Outstanding Reviewer: ICML 2022, NeurIPS 2024 Main Track, NeurIPS 2024 Dataset & Benchmark Track
- 令和四年度 (2022) PRMU研究奨励賞 (outstanding research award at a Japanese domestic conference)
- ACML2023 Best Paper Award